RESEARCH
My independent research focuses on Bayesian nonparametric methods and hierarchical Bayesian models and their applications in large-scale biological sciences. Because Bayesian methods tend to be computationally intensive (especially for large-scale studies), I have also devoted a part of my research to developing more efficient computational methods in order to facilitate the application of Bayesian statistics to data-intensive scientific problems. I am currently focusing on the following areas:
Nonparametric Bayesian models
While parametric models are convenient and easy to interpret, they are constrained by assumptions that rarely hold true in practice. Modern Bayesian nonparametric methods, such as Dirichlet process mixtures (DPM) and Gaussian process (GP) models, liberate quantitative scientists from the shortcomings of assuming simple distributional forms (e.g., normality) and linear relationships among variables. Dirichlet process mixture models are typically used for nonparametric density estimation and clustering. With Radford Neal, we expanded the application of DPM by proposing a novel nonlinear classifier that models the joint distribution of the response and predictor variables nonparametrically using Dirichlet process mixtures. Also, in recent years, my students and I have been using Gaussian processes to develop flexible methods for modeling time series data and identifying relationships among multiple time series.
Scalable Bayesian inference
Massive datasets have created exciting new opportunities yet have imposed new challenges for the scientific community. These new data-intensive problems are especially challenging for Bayesian methods, which typically involve intractable models that rely on computationally intensive simulations for their implementation. While simple algorithms (e.g., random walk Metropolis) might be effective at exploring low-dimensional distributions, they can be very inefficient for complex, high-dimensional distributions. To address this issue, we have been developing methods that exploit the geometric properties of the parameter space to improve the efficiency of sampling algorithms. My students and I have published several papers on variations of Hamiltonian Monte Carlo. In these projects, we have been collaborating with my colleagues Hongkai Zhao and Jeff Streets.
Statistical Methods in Neuroscience
My methodological research is mainly motivated by applied problems. The main focus of my applied research has been on large-scale biological studies. I am mainly interested in analysis of large-scale neurophysiological studies. We have recently developed new statistical models to capture temporal cross-dependencies among multiple neurons by simultaneous modeling of their spike trains using Gaussian processes. In these projects, we are closely collaborating with my colleagues Hernando Ombao, Sam Behseta, and Pierre Baldi, as well as several scientists including Norbert Fortin, Steve Cramer, and David Moorman.
(949) 824-0623
2222 ISEB, UC Irvine, CA 92697
babaks at uci dot edu
Contact

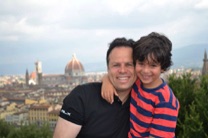
